The Next Wave of AI: Small, Specialized, and Cost-Effective
Dec 18, 2024
Let's be honest: we've been using GPT-4 or Claude Sonnet 3.5 for everything. From complex reasoning tasks to simple text classification, we've been throwing our most powerful (and expensive) model at every problem that comes our way. But something interesting is happening in AI right now. We're finally moving past this one-size-fits-all approach and into the "how do we actually make this work efficiently?" phase. And it's about time.
2024 was all about playing with AI. Everyone was building prototypes, testing capabilities, and generally being amazed by what GPT-4 could do. At StaffAgent.AI, we saw firsthand how AI could transform recruitment by conducting initial candidate interviews. But as we look ahead to 2025? That's when things are getting serious.
The Real Problem with AI in Production
Here's the thing about running AI in production: it's expensive. Really expensive. Take GPT-4. It costs about $4 per million tokens. That might not sound like much until you're processing thousands of interviews per month, analyzing responses, and generating detailed candidate assessments.
The obvious solution seems to be using smaller models like GPT-4 Mini, which costs 15 times less. But then you hit another problem: these smaller models aren't as smart. They make more mistakes. They miss nuances. When you're evaluating candidates, you can't afford these mistakes.
So you're stuck between a rock and a hard place: pay through the nose for GPT-4, or save money but deliver a worse experience.
Enter Distillation
But there's a way out. It's called distillation, and it's basically like teaching a smaller model to be smart about one specific thing.
Think about it this way: GPT-4 is like a senior recruiter who knows everything about every industry and role. That's great, but you're paying senior-level rates for every task. What if instead, you could train a specialist to be really good at just the one thing you need?
That's distillation. You take GPT-4's knowledge about your specific task - maybe it's analyzing communication skills in interviews, or evaluating technical responses - and you transfer that knowledge to a smaller, cheaper model.
Here's How It Actually Works
First, you collect examples of GPT-4 doing your specific task really well
Then, you use these examples to train a smaller model
Finally, you test the smaller model to make sure it learned the right things

The results can be surprising. In OpenAI's demo, they took a task where GPT-4 scored 91% accuracy. The small model initially scored 76% - pretty terrible. But after distillation? 88%. Nearly as good as GPT-4, at a fraction of the cost.
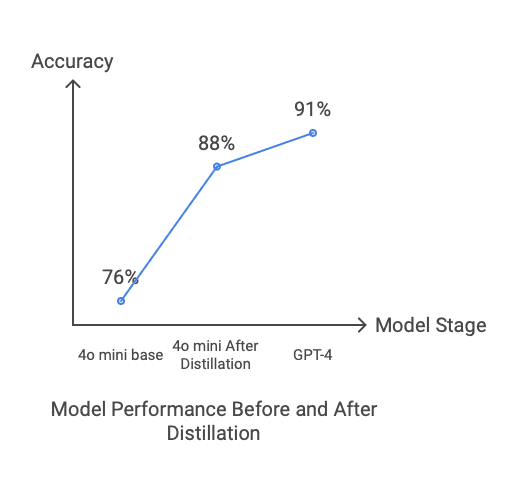
But It's Not Magic
Distillation isn't perfect for everything. It works best when:
Your task is focused and specific (like sentiment analysis or skill assessment)
You don't need the model to reason about complex, novel situations
You have good, representative data to train with
It struggles with tasks that require broad knowledge or perfect precision. You wouldn't use it to build a general-purpose chatbot or handle complex edge cases in candidate evaluation.
The Future is Many Models
Here's what's really interesting: the future of AI applications isn't going to be one big model doing everything. It's going to be dozens of smaller, specialized models working together.
Think about it like your recruitment team. You don't have one person who does everything. You have specialists. Your AI should work the same way:
Small, distilled models for specific, routine tasks like initial screening
Big models for complex, reasoning-heavy work like final assessments
Each piece doing what it does best, at the right price point
The Bottom Line
If you're running AI in production, you need to be thinking about distillation. It's not just about saving money - though that's nice. It's about building sustainable AI systems that can actually scale with your business.
The era of throwing GPT-4 at every problem is ending. The future is smarter, smaller, and more specialized. And that's a good thing.
Here is the full video that explains distillation process and a nice demo.